Active Projects
These are projects that are very actively under development.
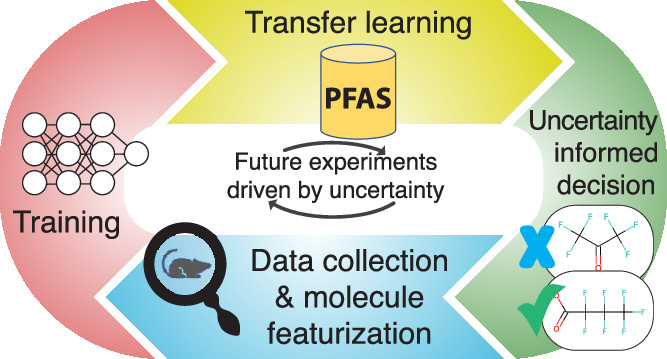
AI4PFAS
Deep learning workflow and dataset for toxicity prediction.
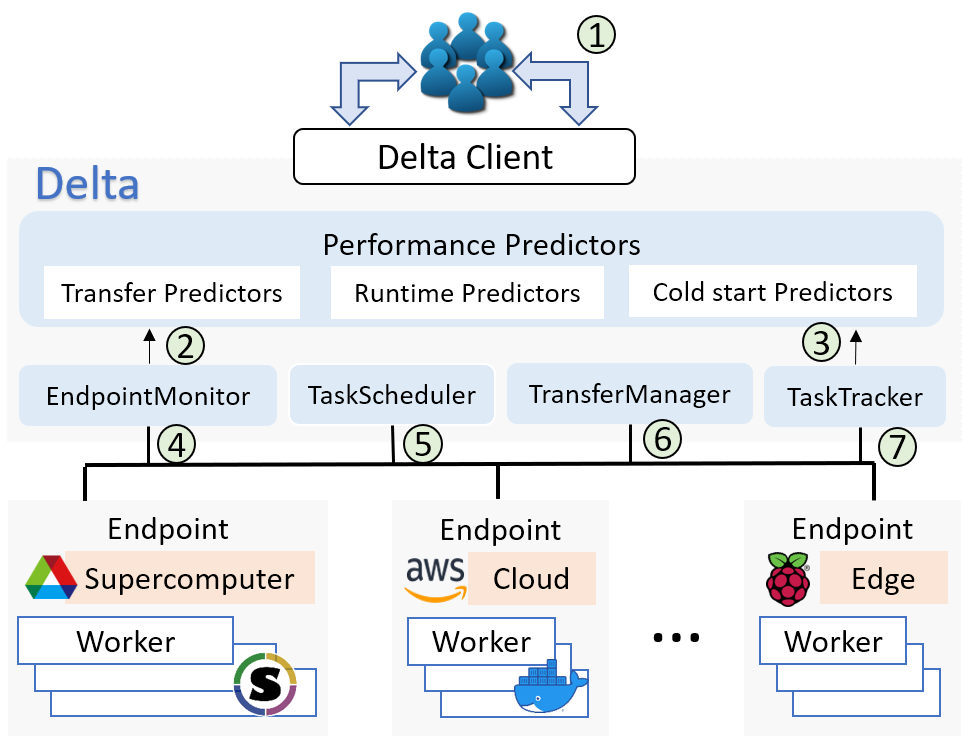
Cost-aware computing
Automating cost-aware profiling, prediction, and provisioning of cloud and HPC resources.
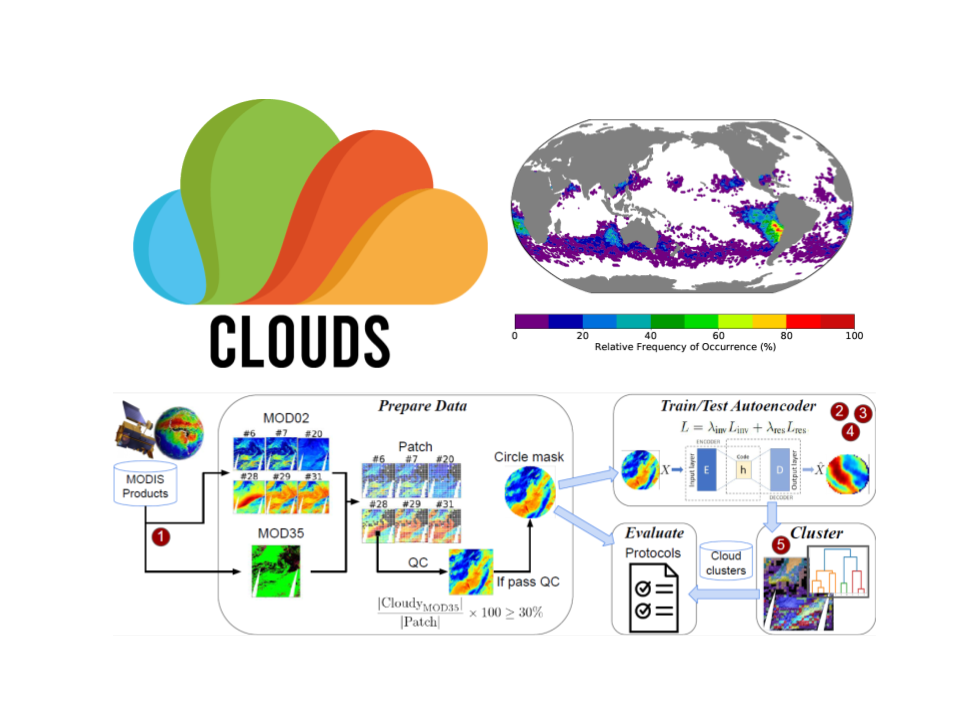
Clouds
Self-supervised data-driven methods for the application on big satellite clouds imagery
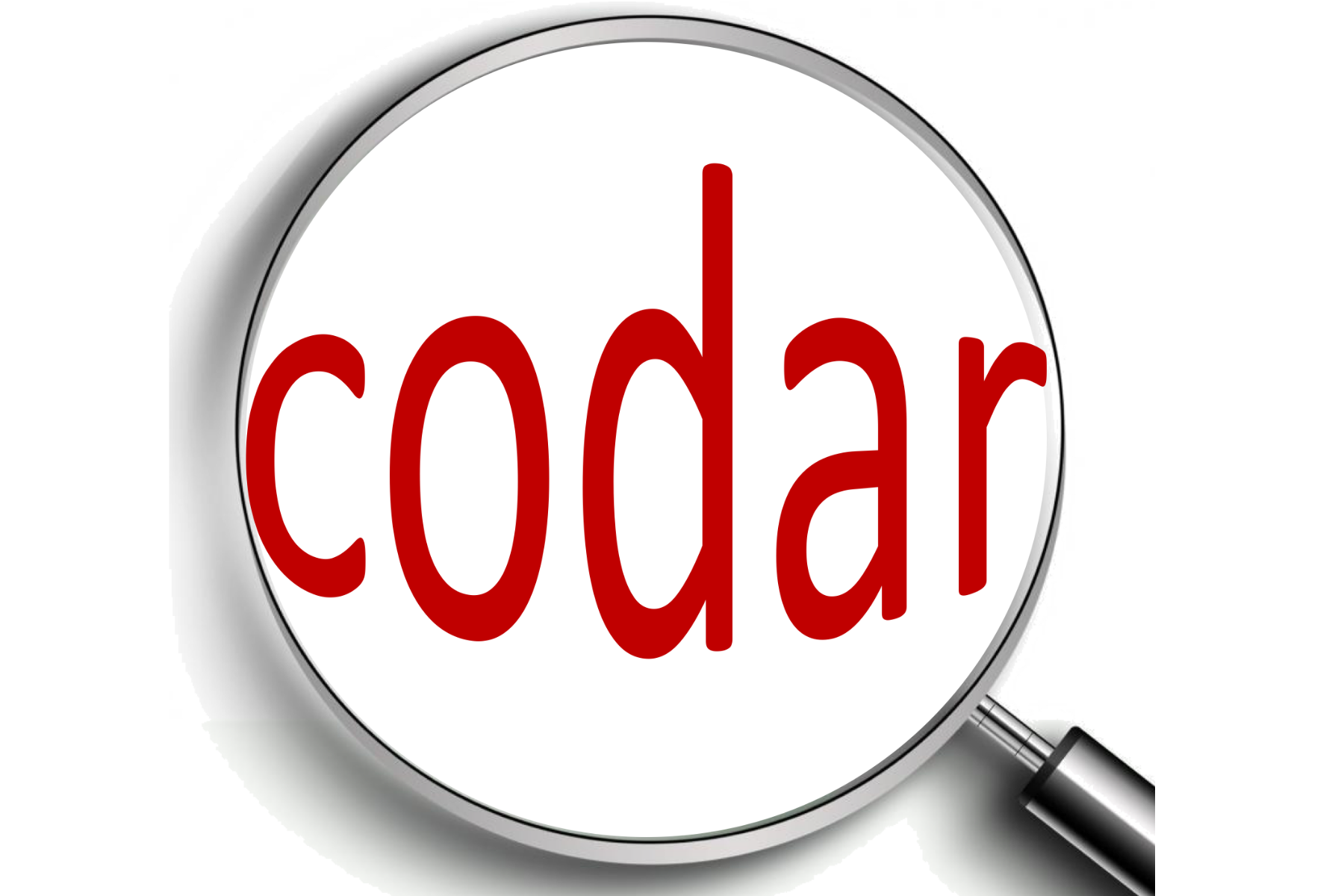
CODAR
We develop methods for online data analysis and reduction on exascale computers
Colmena
Machine Learning-Based Steering of Ensemble Simulations
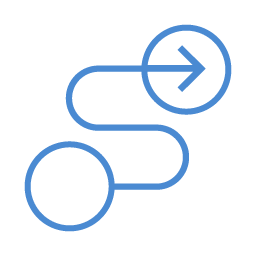
Flows
We are developing methods to automate the scientific data lifecycle.
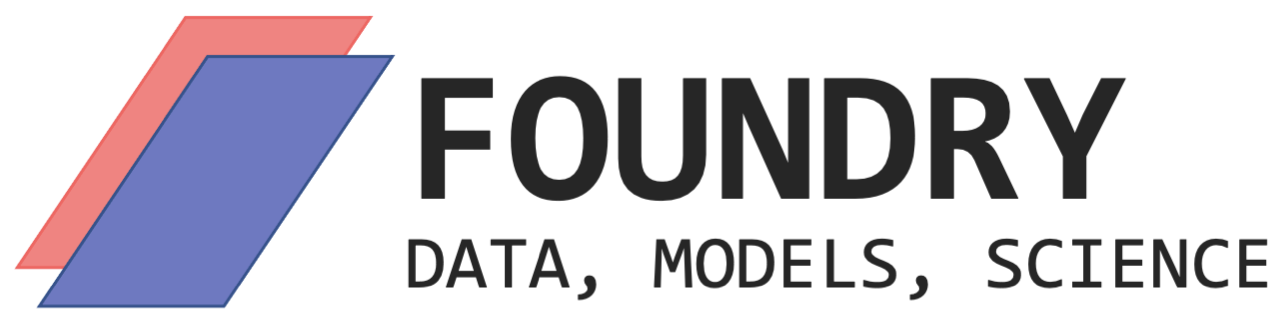
Foundry
An open source machine learning platform for scientists
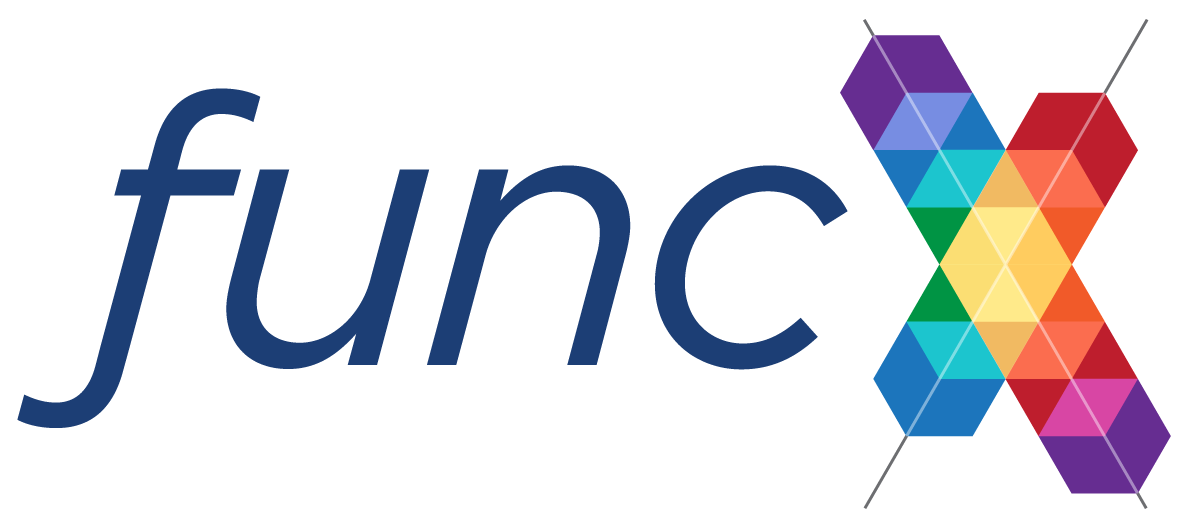
funcX
funcX is a Function as a Service platform for scientific computing.
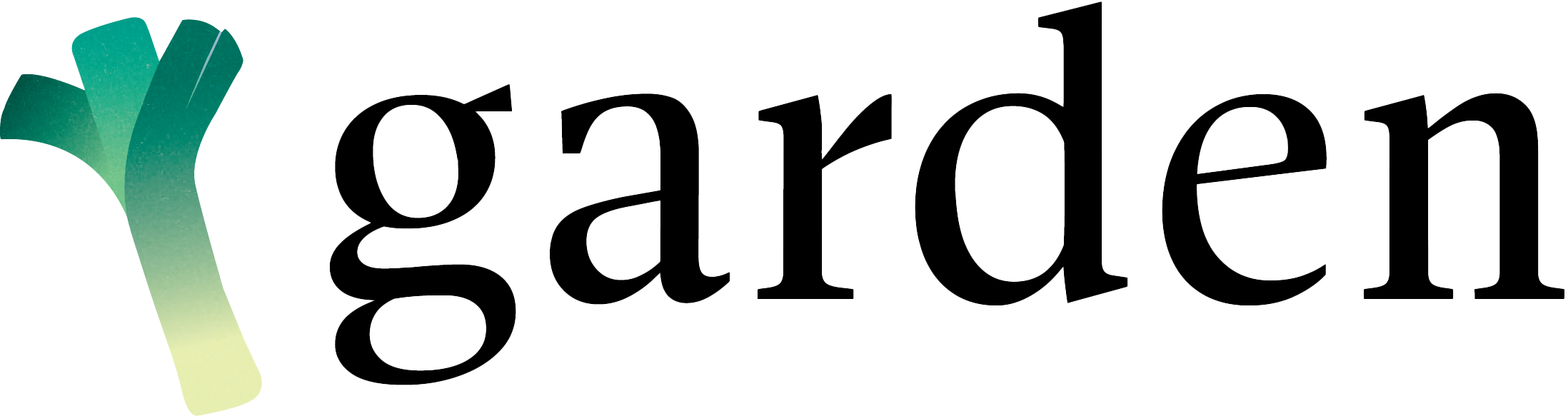
Garden
Garden turns researchers' AI models into citable APIs that run on scientific computing infrastructure.
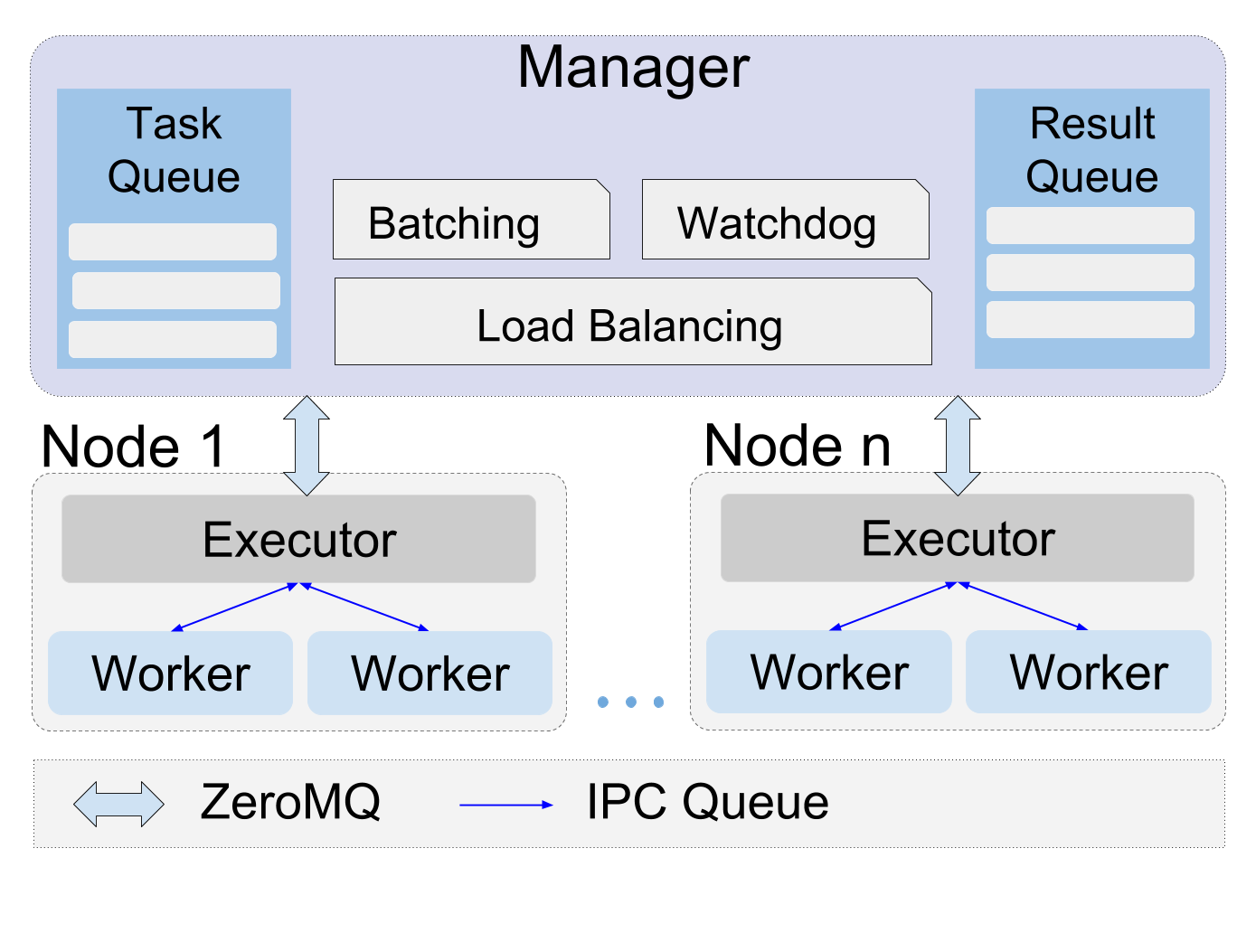
Gladier
Globus Automation for Data-Intensive Experimental Research
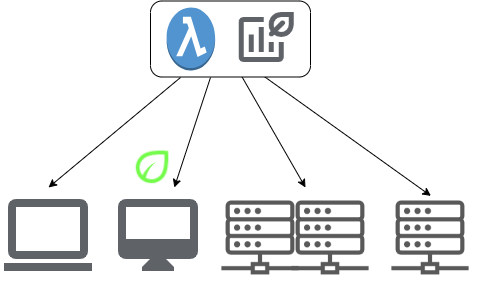
GreenFaaS
A monitoring and scheduling framework to find the most energy-efficient endpoints for your application.
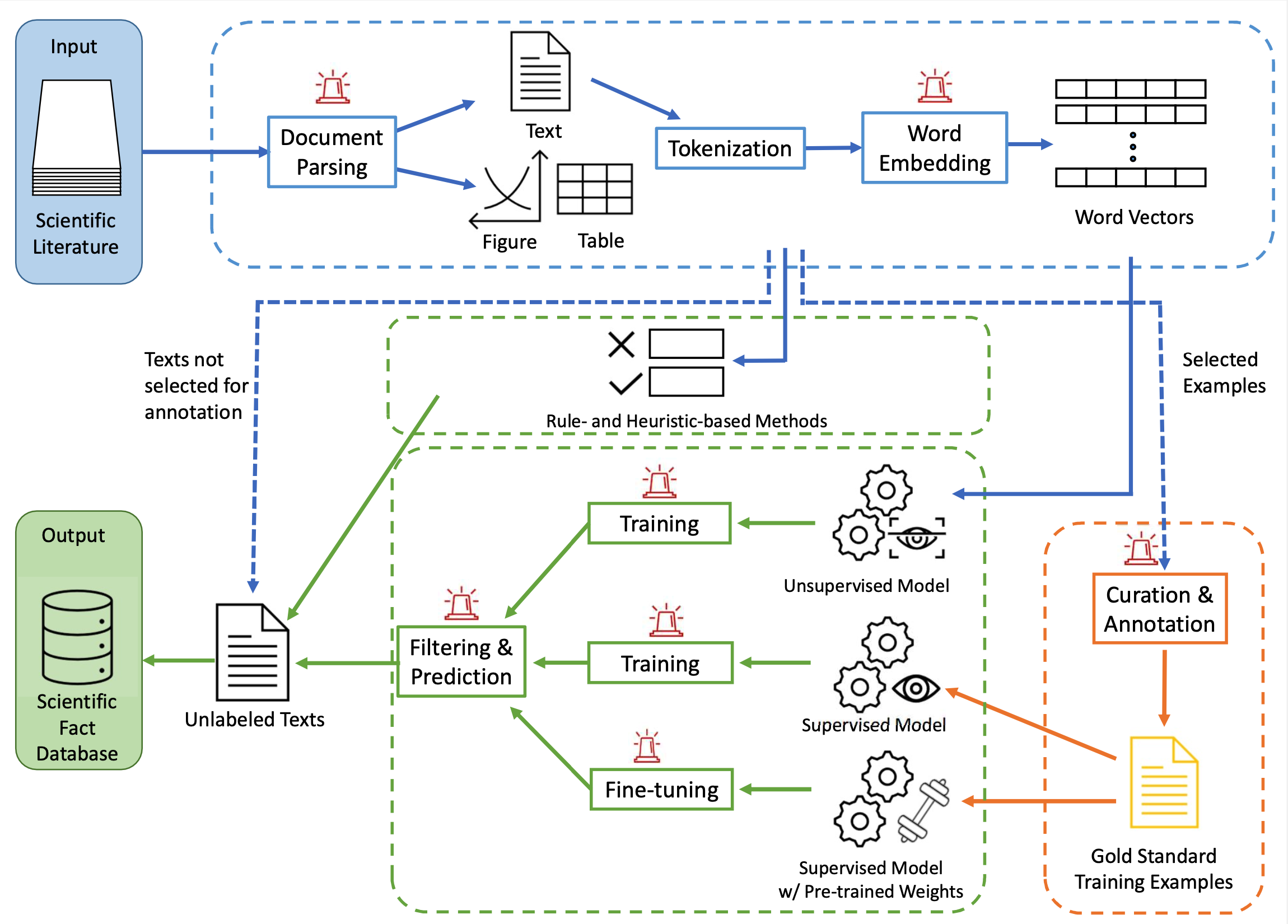
Scientific Language Modeling and Information Extraction
Data mining from literature with a foundational science-focused language model
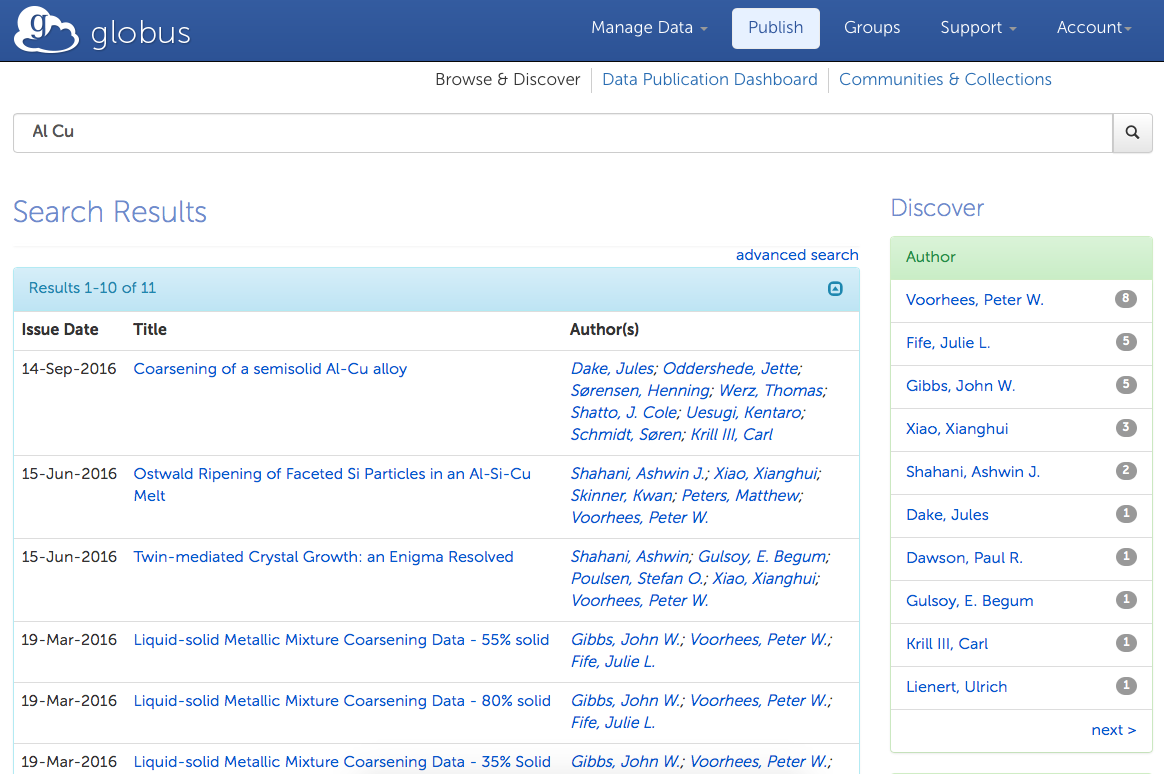
The Materials Data Facility
We are creating data services to help materials scientists publish and discover data
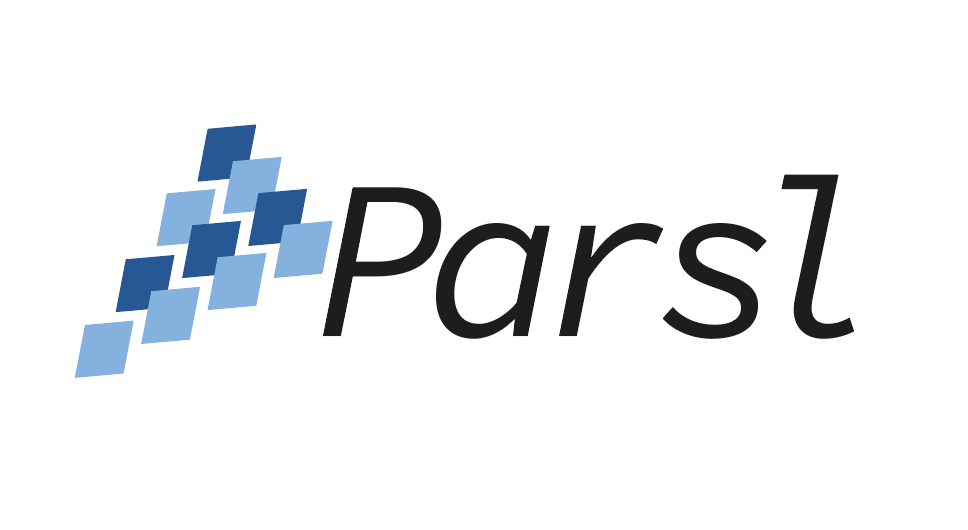
Parsl
Parallel programming library for Python
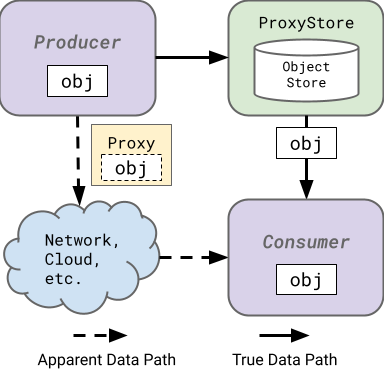
ProxyStore
Facilitate efficient data flow management in distributed Python applications.
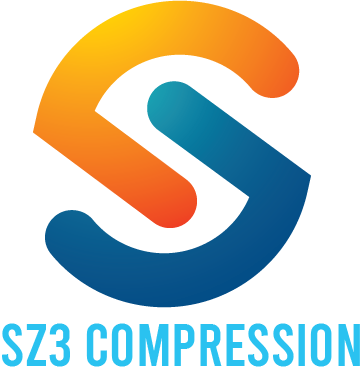
SZ3 Compression
We develop a series of prediction-based lossy compression algorithms for scientific simulations.
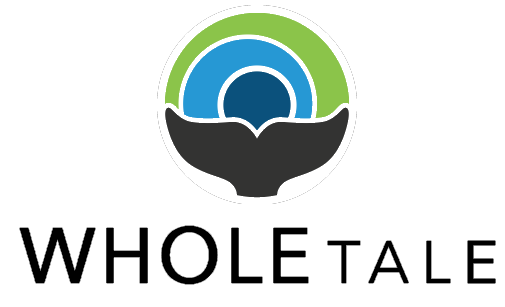
Whole Tale
Whole Tale is a cloud-hosted platform for conducting and sharing reproducible science
Other Projects
These are projects that are not currently being actively developed but may or may not still be used to support above active projects.
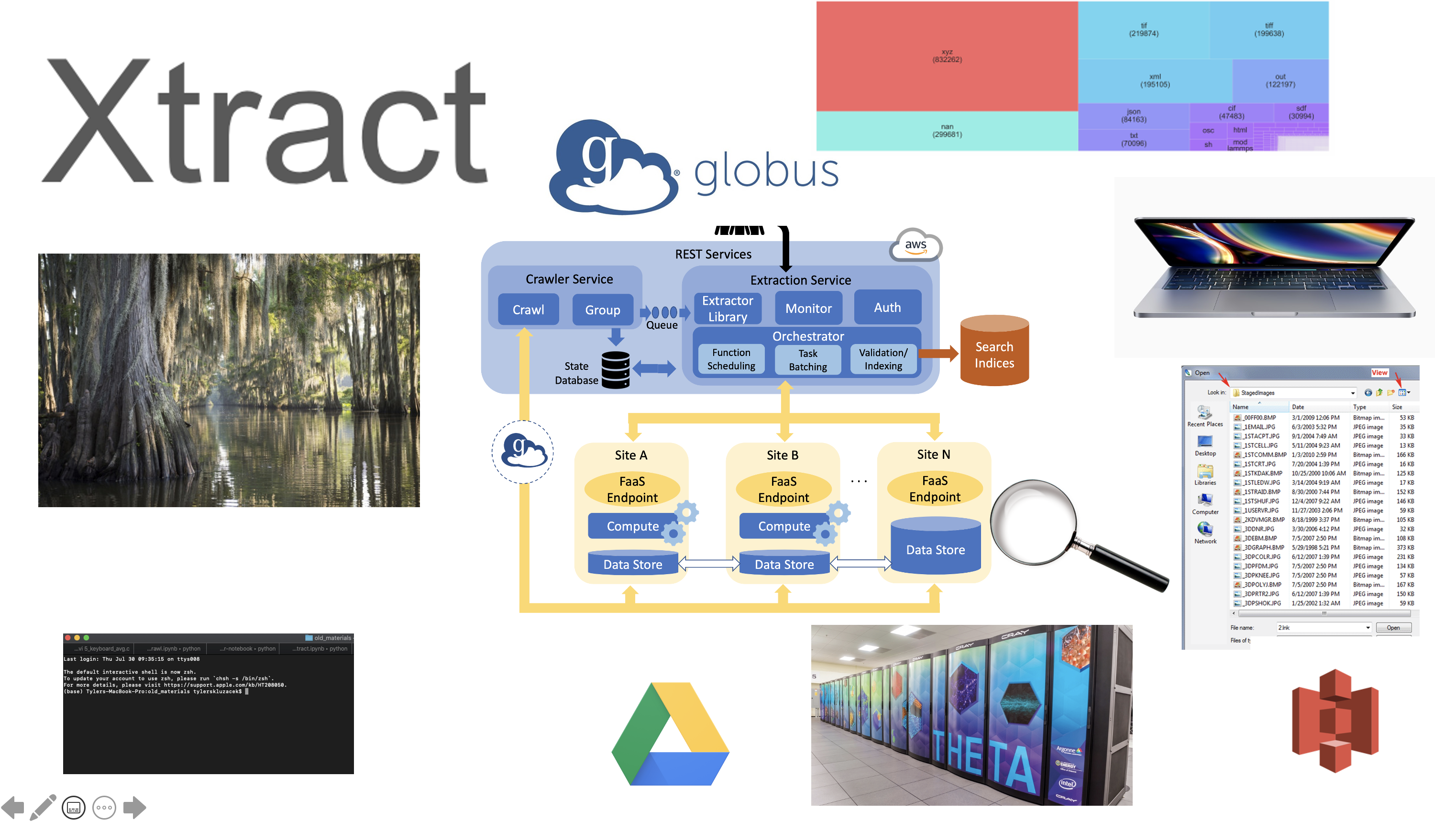
Xtract
Metadata Extraction for Everyone
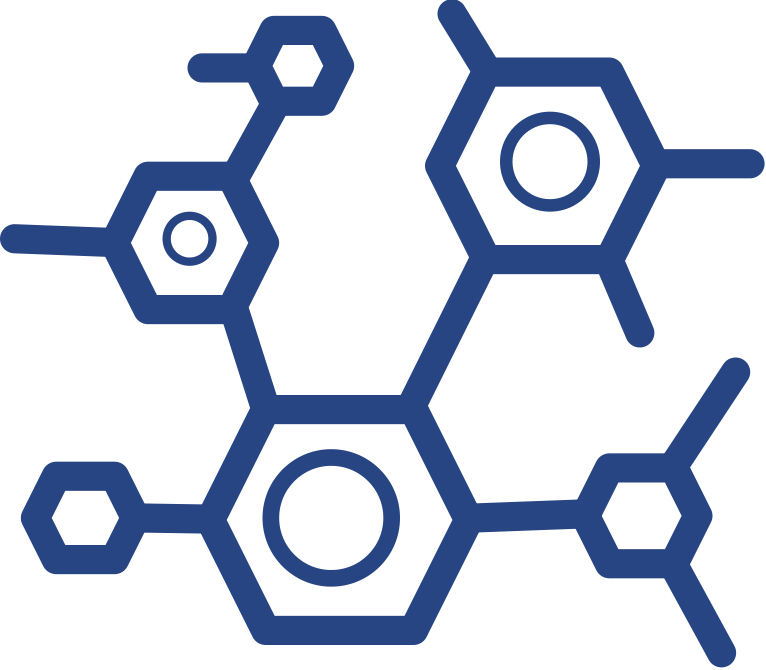
Data and Learning Hub for Science (DLHub)
A simple way to find, share, publish, and run machine learning models and discover training data for science
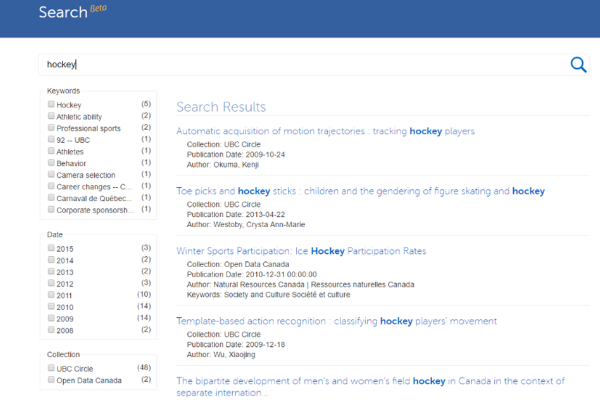
Globus Search
We are developing methods to index large amounts of scientific data distributed over heterogeneous storage systems
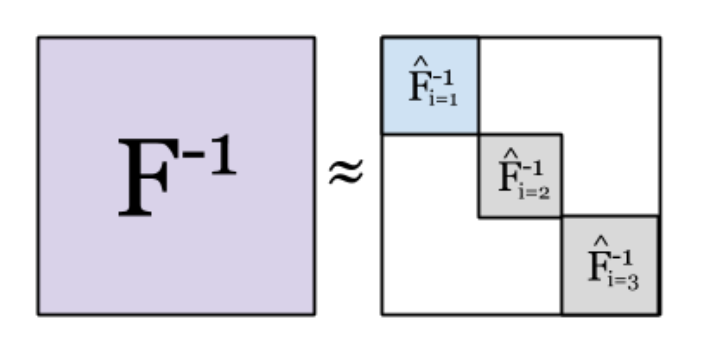
KAISA: Scalable Second-Order Deep Neural Network Training
KAISA is a novel distributed framework for training large models with K-FAC at scale.