Globus Labs is a research group led by Prof. Ian Foster and Dr. Kyle Chard that spans the Department of Computer Science at the University of Chicago and the Data Science and Learning Division at Argonne National Laboratory. Our modest goal is to realize a world in which all research data are reliably, rapidly, and securely accessible, discoverable, and usable. To this end, we work on a broad range of research problems in data-intensive computing and research data management. Our work is made possible by much-appreciated support from the National Science Foundation, National Institutes of Health, Department of Energy, National Institute of Standards and Technology, and other sources, and in addition to computer science, engages fields as diverse as materials science, biology, archaeology, climate policy, and social sciences. We work closely with the team developing the Globus research data management platform, who often challenge us to think bigger—and sometimes implement our less crazy ideas.
Featured Projects
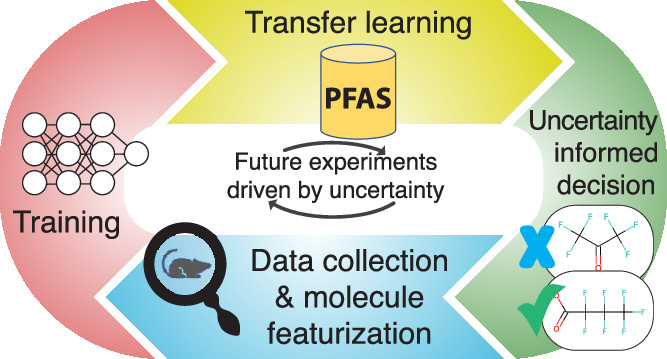
AI4PFAS
Deep learning workflow and dataset for toxicity prediction.
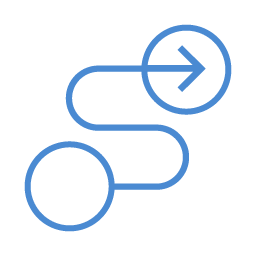
Flows
We are developing methods to automate the scientific data lifecycle.
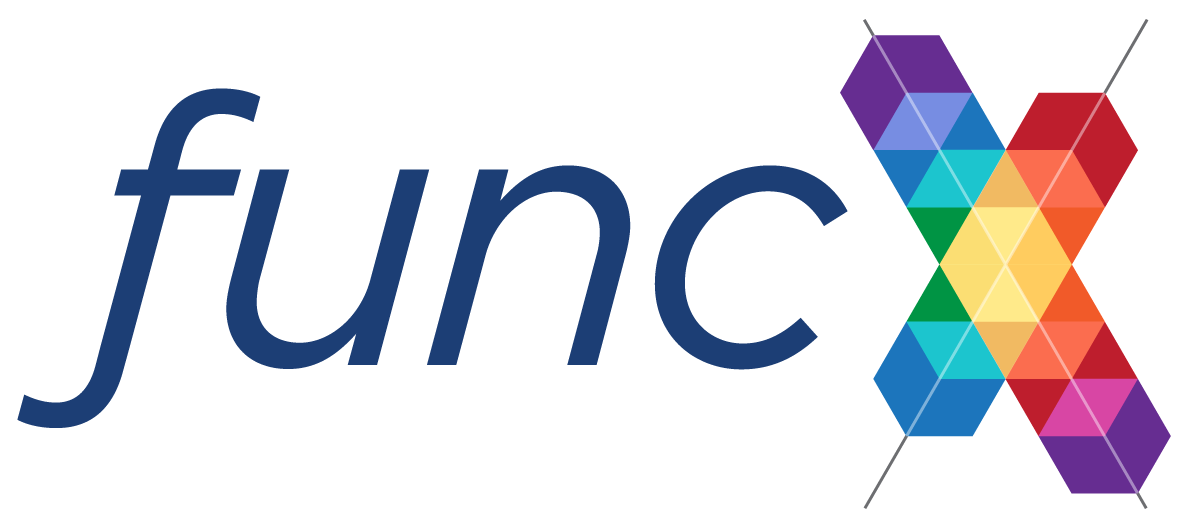
funcX
funcX is a Function as a Service platform for scientific computing.
Recent News
Ian accepted his ACM Ken Kennedy Award and gave his Ken Kennedy Lecture at SC22!
Congrats to Greg, Valerie, Logan, Ian, and collaborators for winning the Gordon Bell Special Price for COVID-19 Research at SC22! Check out the prize-winning paper: “GenSLMs: Genome-Scale Language Models Reveal SARS-CoV-2 Evolutionary Dynamics”! Several other GLabs projects were used in this work, including Parsl, Colmena, and ProxyStore!