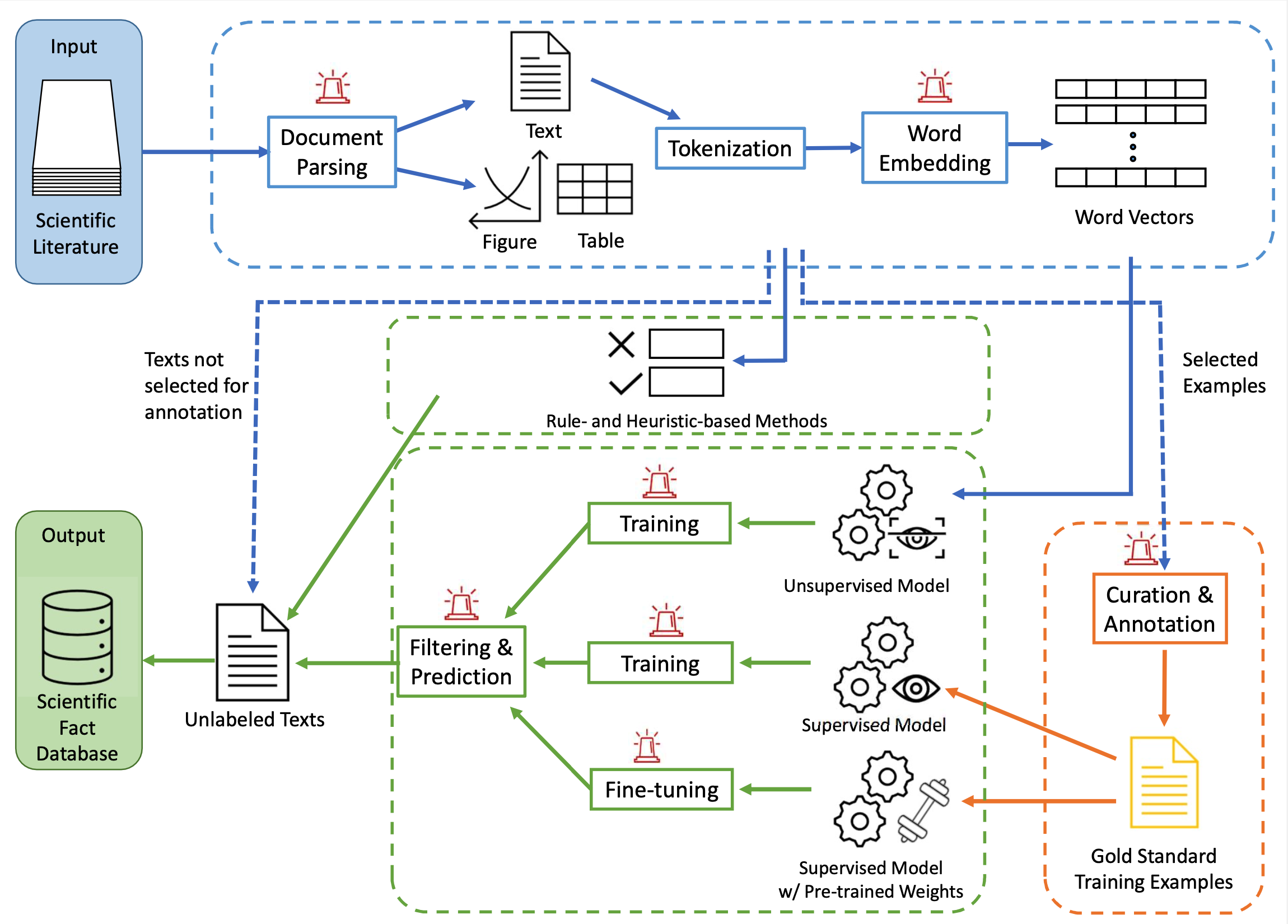
Tags
#Machine Learning #Materials ScienceScientific Language Modeling and Information Extraction
Scientific articles have long been the primary means of disseminating scientific discoveries. Valuable data and potentially ground-breaking insights have been collected and buried deep in the mountain of scientific publications over the centuries. We strive to answer interesting and important questions in science by extracting facts from publications and, in the process, have built a foundational large language model for science.
Our previous works have focused on designing application-specific models and pipelines, which has produced a polymer extraction model that outperformed a leading chemical extraction toolkit by up to 50%, as measured by F1 score, as well as a druglike molecule extraction model that found 3,591 molecules from COVID-19-related medical research that had not been previously considered by Argonne’s computational screening research team.
Large Language Models (LLMs) has become of core of many NLP solutions in recent years due to their flexibility and performance advantages over traditional machine learning methods. Most publicly available pretrained LLMs are pretrained on general English corpora such as news reports, wiki pages, and blogs, while scientific texts are usually neglected. We have pretrained on the ScholarBERT and ScholarBERT-XL models on a corpus of 75 million of scientific publications. Preliminary experimental results showed their strengths in identifying disciplines corresponding to scientific named entities compared to general LLMs or domain-specific LLMs.
Publications
- Zhi Hong, J. Gregory Pauloski, Aswathy Ajith, Eamon Duede, Kyle Chard, and Ian Foster “Accelerating Computational Social Science with ScholarBERT.” 8th International Conference on Computational Social Science, 2022.
- Zhi Hong, Aswathy Ajith, Gregory Pauloski, Eamon Duede, Carl Malamud, Roger Magoulas, Kyle Chard, and Ian Foster. ”ScholarBERT: Bigger is Not Always Better.” arXiv preprint arXiv:2205.11342 (2022
- Zhi Hong, Logan Ward, Kyle Chard, Ben Blaiszik, and Ian Foster. “Challenges and Advances in Information Extraction From Scientific Literature: A Review.” Accepted to The Journal of The Minerals, Metals \& Materials Society (JOM). 2021.
- Zhi Hong, J. Gregory Pauloski, Logan Ward, Kyle Chard, Ben Blaiszik, and Ian Foster. “Models and Processes to Extract Drug-like Molecules from Natural Language Text.” Frontiers in Molecular Biosciences: 826. 2021.
- Zhi Hong, Roselyne Tchoua, Kyle Chard, Ian Foster, “SciNER: Extracting Named Entities from Scientific Literature.”. International Conference on Computational Science, 308-321. 2020.
- Tchoua, Roselyne, Zhi Hong, Debra Audus, Shrayesh Patel, Logan Ward, Kyle Chard, Juan De Pablo, and Ian Foster. “Developing databases for polymer informatics.” Bulletin of the American Physical Society 65 (2020).
Funding and Acknowledgements
This work was performed under award 70NANB19H005 from U.S. Department of Commerce, National Institute of Standards and Technology as part of the Center for Hierarchical Materials Design (CHiMaD); by the U.S. Department of Energy under contract DE-AC02-06CH11357; and by U.S. National Science Foundation awards DGE-2022023 and OAC-2106661. This research used resources of the University of Chicago Research Computing Center and the Argonne Leadership Computing Facility, a DOE Office of Science User Facility supported under Contract DE-AC02-06CH11357.